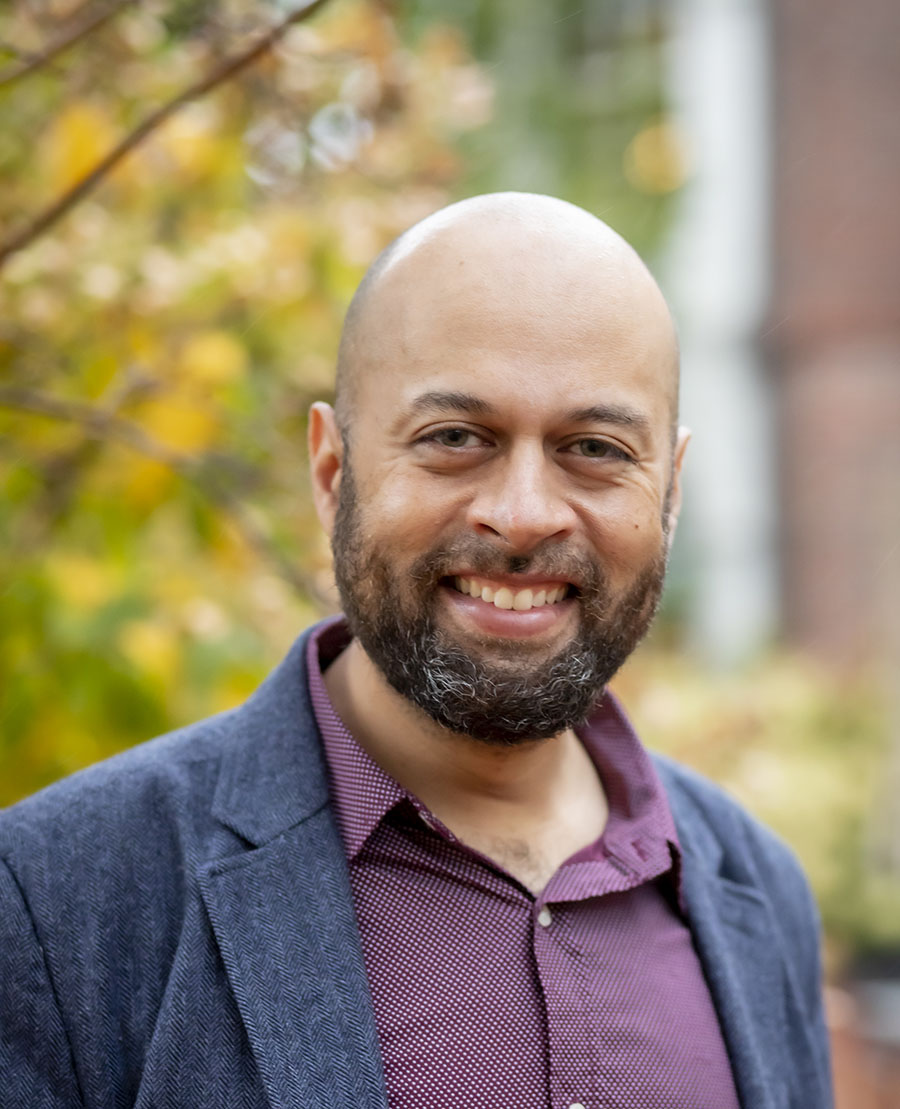
Carlos Ponce, M.D.,Ph.D.
The Neuroscience of Visual Recognition and Perception
Our main goal is to understand how the visual brain works in the natural world, when it is presented with rich and complex scenes, such as those we encounter in our daily lives.
We focus our research on the brain of the rhesus macaque, employing electrophysiological techniques to record neuronal activity across the visual cortex hierarchy, including areas V1, V2, V4, inferotemporal cortex, and prefrontal cortex. Our approaches use leading-edge neural networks, both as tools for generating stimuli (e.g., generative adversarial networks) and as models for the visual system itself (e.g., convolutional neural networks, vision transformers). These powerful, machine-intelligence models enable us to manipulate images in complex ways, and to identify the types of visual information encoded by cortical neurons. We compare this information with that encoded by different neural network architectures, while also leveraging monkey behavior to gain insights into the origins and evolution of visual representations.
Behavioral tasks play a key role in our research. Thus, we are continually refining our animal training techniques, incorporating computer-based automation and tablet-based training directly within the animals' home cages. Our methodologies are grounded in ethological and ethical principles, allowing us to adjust the complexity of tasks to the animals' natural capabilities.
By bridging visual neuroscience and machine learning, we hope to advance automated visual recognition technologies in areas like medical imaging, security, and autonomous driving, and also shed light on the subjective experience of vision itself.
International Conference on Learning Representations